Thermodynamic Computing: The Next Frontier in AI Hardware
As artificial intelligence (AI) continues to advance, the need for more efficient and powerful computing hardware is becoming a pressing issue. Traditional silicon-based chips are reaching their physical and energy efficiency limits, making it essential to explore alternative computing models. One such promising field is thermodynamic computing, which leverages the laws of thermodynamics to perform computations in a radically new way. This article explores what thermodynamic computing is, its advantages, and its potential impact on AI hardware.
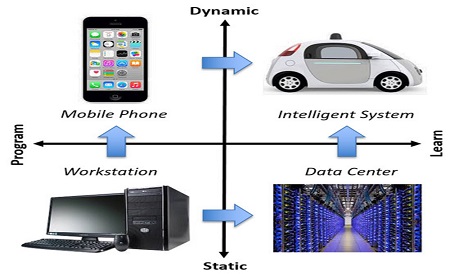
What is Thermodynamic Computing?
Thermodynamic computing is an emerging approach to computation that utilizes energy fluctuations, entropy, and the probabilistic nature of thermodynamic systems to process information. Unlike conventional binary computing, which relies on a strict on/off state (0s and 1s), thermodynamic computing takes advantage of natural energy dissipation and probabilistic states to perform calculations.
How Thermodynamic Computing Works
At its core, thermodynamic computing is inspired by the way nature processes information. It operates by encoding information in the energy states of a physical system and allowing natural thermal fluctuations to guide computations. This concept is particularly effective in tasks where probabilistic reasoning and massive parallel processing are required, such as AI and deep learning.
Advantages of Thermodynamic Computing
Compared to traditional computing architectures, thermodynamic computing offers several unique advantages:
- Energy Efficiency: Since it relies on naturally occurring thermodynamic processes, this approach could significantly reduce energy consumption compared to traditional CPUs and GPUs.
- Parallel Processing: Thermodynamic systems inherently support parallel computation, making them ideal for AI workloads that require large-scale data processing.
- Beyond Moore’s Law: As transistor miniaturization reaches its physical limits, thermodynamic computing provides a new path for continued performance improvements without relying on silicon scaling.
- Better AI Performance: Probabilistic computing models are particularly well-suited for AI tasks like machine learning, pattern recognition, and neural network optimization.
Challenges and Future of Thermodynamic Computing
Despite its potential, thermodynamic computing is still in its infancy. Some of the key challenges include:
- Implementation Complexity: Building hardware capable of leveraging thermodynamic principles for practical computing is still a significant engineering challenge.
- Industry Adoption: Major tech companies and AI researchers need to invest heavily in this field before it can become commercially viable.
- Software Adaptation: Current AI models are designed for classical computing architectures, meaning new software paradigms will be needed to fully utilize thermodynamic computing.
Conclusion
Thermodynamic computing represents a bold new frontier in AI hardware, offering the promise of faster, more efficient, and fundamentally different computing architectures. While challenges remain, ongoing research and investment in this field could revolutionize how AI processes information in the coming years.